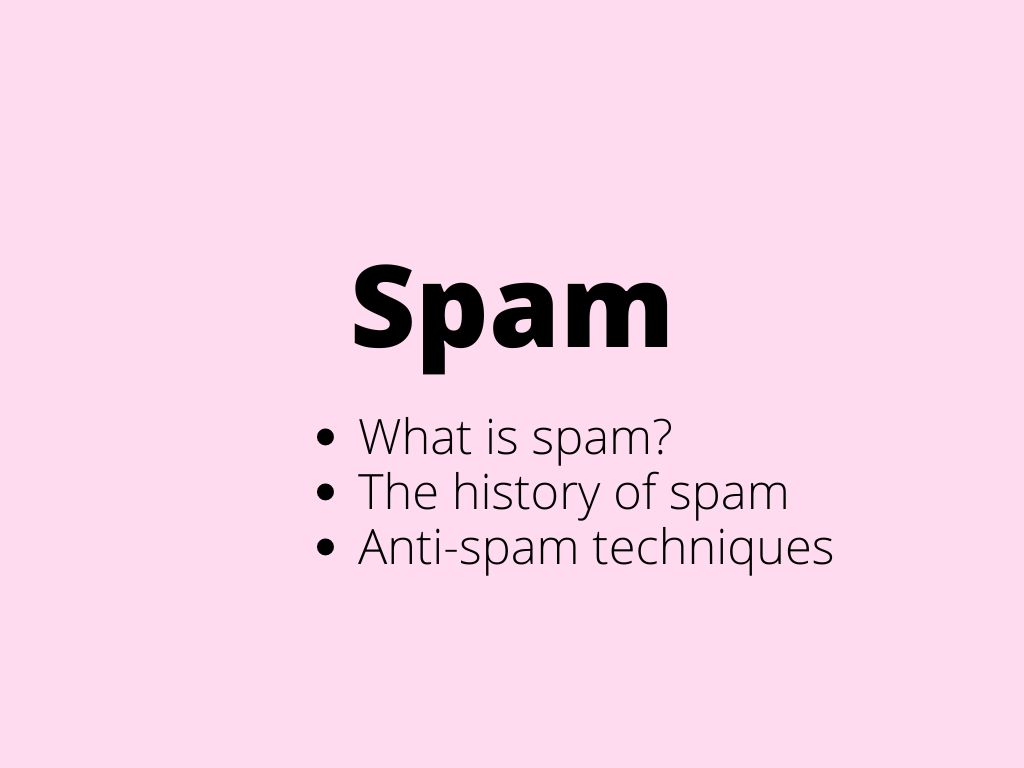
Spam – what is it?
Spam, in the context of electronic communication, refers to unsolicited, often irrelevant messages sent over the internet, typically to a large number of users for advertising, phishing, spreading malware, or other malicious purposes. The term “spam” has evolved significantly since its inception, both in its definition and its impact on digital communication. This article delves into the origins, evolution, impact, and countermeasures associated with spam.
Origin of the term “spam”
The word “spam” originally comes from a brand of canned precooked meat made by Hormel Foods. Its association with unsolicited electronic messages can be traced back to a Monty Python sketch from 1970, in which the word “spam” is repeatedly sung, drowning out other conversations. Early internet users adopted the term to describe unwanted messages that overwhelmed useful communication, much like the repetitive chant in the sketch.
Early days of spam – the first spam message
The first documented instance of electronic spam occurred in 1978 when Gary Thuerk, a marketer for Digital Equipment Corporation (DEC), sent an unsolicited email to approximately 400 users on ARPANET. This email advertised a product demonstration and is considered the first spam email. Although it generated interest and resulted in some sales, it also sparked considerable backlash and highlighted the potential for unsolicited messages to disrupt communication.
Usenet spam
In the early 1990s, spam began to proliferate on Usenet newsgroups, a precursor to modern Internet forums. The most infamous early spam on Usenet occurred in 1994 when lawyers Laurence Canter and Martha Siegel posted an advertisement for their immigration law services to thousands of newsgroups, irrespective of their relevance. This incident, known as the “Green Card Lottery” spam, led to widespread outrage and efforts to control such practices.
The “Green Card Lottery” spam incident
One of the most infamous early instances of spam on Usenet occurred in 1994. Lawyers Laurence Canter and Martha Siegel posted an advertisement for their immigration law services to thousands of newsgroups, regardless of their relevance. This incident became known as the “Green Card Lottery” spam and led to widespread outrage among Usenet users.
The “Green Card Lottery” spam incident highlighted the disruptive potential of unsolicited messages on digital platforms. It led to increased efforts to control such practices, including the development of spam filters and community guidelines to manage and mitigate spam.
Email spam
With the rise of email as a primary mode of communication, spam emails became increasingly prevalent. Spammers exploited the open nature of email protocols to send vast quantities of unsolicited messages. The low cost and ease of sending emails made it an attractive medium for advertisers and scammers. By the late 1990s, email spam had become a significant problem, leading to the development of various filtering technologies and legislative measures.
The problem of email spam
By the late 1990s, email spam had become a significant problem for users and service providers alike. The sheer volume of spam emails began to overwhelm inboxes, making it difficult for users to find legitimate messages. The proliferation of spam also posed security risks, as many spam emails contained phishing attempts or malware.
Technological responses to email spam
To combat the growing problem of email spam, various filtering technologies were developed. These included:
- Content filters – analyzing the content of emails to identify and filter out spam based on keywords and patterns.
- Blacklists – maintaining lists of known spam sources and blocking emails from those sources.
- Bayesian filters – using statistical methods to identify spam based on the probability of certain words and phrases appearing in spam emails.
Content filters – how we can analyze email content to identify and filter out spam
Content filters are a crucial component of email security, designed to analyze the content of emails to identify and filter out spam based on keywords and patterns. These filters play a significant role in protecting users from unwanted and potentially harmful emails.
How content filters work
Content filters function by examining various elements within an email, including the subject line, body text, attachments, and even metadata. Here’s a detailed look at the key processes and techniques involved:
- Keyword analysis – content filters scan emails for specific keywords or phrases commonly associated with spam. These keywords can include terms related to financial scams, adult content, or promotional language. For instance, words like “free,” “urgent,” and “guaranteed” are often flagged as spam indicators.
- Pattern recognition – beyond keywords, content filters use pattern recognition to detect suspicious email structures. This involves analyzing the frequency and placement of certain elements, such as hyperlinks, images, and repeated phrases. Patterns commonly found in spam emails are used to develop rules that help identify similar messages in the future.
- Statistical techniques – advanced content filters employ statistical models to evaluate the likelihood that an email is spam. Bayesian filtering, for example, calculates the probability of an email being spam based on the presence of specific words and patterns. This method continually improves its accuracy by learning from past emails marked as spam or legitimate.
- Heuristic analysis – heuristic analysis involves using predefined rules to assess the characteristics of an email. This includes evaluating the email’s structure, formatting, and language. Emails with excessive capitalization, misspellings, or unusual formatting are often flagged as spam.
- Machine learning – modern content filters leverage machine learning algorithms to enhance their detection capabilities. These algorithms analyze vast amounts of data to identify subtle patterns and anomalies associated with spam. Machine learning models can adapt over time, improving their ability to distinguish between spam and legitimate emails.
Benefits of content filters
Implementing content filters offers several advantages in the fight against spam:
- Improved email security – by filtering out spam, content filters protect users from phishing attacks, malware, and other malicious content that can compromise security.
- Enhanced productivity – reducing the volume of spam emails allows users to focus on important communications, improving overall productivity.
- Cost savings – efficient spam filtering reduces the need for additional resources to manage and mitigate the effects of spam, leading to cost savings for organizations.
The main challenges in content filtering
Despite their effectiveness, content filters face several challenges:
- Evasion techniques – spammers continuously develop new tactics to evade content filters. These include obfuscation, where keywords are deliberately misspelled, and using images to convey spammy content, which text-based filters might not detect.
- False positives and negatives – content filters must balance the risk of false positives (legitimate emails being marked as spam) and false negatives (spam emails bypassing the filter). Striking this balance requires constant tuning and updates to filtering rules.
- Dynamic content – spam content evolves rapidly, necessitating frequent updates to filtering algorithms and rules to maintain effectiveness.
- Resource intensive – analyzing large volumes of email content can be resource-intensive, requiring robust computing power and storage capacity.
Future of content filtering
The future of content filtering will likely see further advancements driven by artificial intelligence and machine learning. These technologies will enhance the ability of filters to adapt to new spam tactics in real time, reducing the incidence of false positives and negatives.
- AI and machine learning – leveraging AI and machine learning will enable more sophisticated pattern recognition and anomaly detection, making filters more effective and efficient.
- Integration with other security measures – content filters will increasingly integrate with broader security frameworks, providing a multi-layered defense against spam and other cyber threats.
- Real-time adaptation – future content filters will be capable of real-time adaptation, continuously learning from new threats and updating their algorithms to stay ahead of spammers.
Spam blacklists – why are blacklists so important nowadays?
Blacklists play a crucial role in the fight against spam by maintaining lists of known spam sources and blocking emails from those sources. This method has become an integral part of email security systems, helping to protect users from unwanted and potentially harmful messages.
How blacklists work
Blacklists function by maintaining databases of IP addresses, domains, and email addresses that are known or suspected to be sources of spam. These lists are used by email servers and security software to filter incoming messages and block those that originate from blacklisted sources. Here’s a detailed look at the key aspects of how blacklists operate:
- Compilation of blacklists – blacklists are compiled using data from various sources, including spam reports from users, spam traps, and intelligence gathered by cybersecurity firms. Spam traps are email addresses created specifically to attract spam, helping identify sources of unsolicited emails.
- Real-time blacklists (RBLs) – these are dynamically updated blacklists that provide real-time data on spam sources. Email servers query these lists to check the reputation of IP addresses and domains before accepting incoming emails.
- DNS-based blacklists (DNSBLs) – these blacklists use the Domain Name System (DNS) to distribute the list of blacklisted IP addresses. When an email server receives a message, it checks the sender’s IP address against the DNSBL. If the IP is listed, the email is rejected or flagged as spam.
- Email blacklists – specific email addresses known to send spam are added to blacklists. These are often addresses associated with phishing, scams, and other malicious activities.
- Domain blacklists – entire domains that have been identified as sources of spam are blacklisted. This includes domains used in email addresses, as well as those hosting malicious websites linked in spam emails.
- IP blacklists – IP addresses that have been used to send spam are added to blacklists. This is particularly useful for blocking bulk spam sent from specific servers or networks.
You can learn more about the term “blacklist” in our previous article Blacklist/blacklists – what is that?, where you can find much more detailed information. Also article about newsletters could help you more understand how spam filters work and what to avoid doing when creating your first newsletter.
Benefits of blacklists
Implementing blacklists offers several advantages in protecting email systems from spam:
- Reduced spam volume – by blocking known spam sources, blacklists significantly reduce the volume of spam that reaches users’ inboxes, enhancing productivity and security.
- Enhanced security – blocking emails from known malicious sources helps prevent phishing attacks, malware distribution, and other cyber threats that can compromise sensitive information.
- Improved user experience – users experience less frustration and wasted time dealing with spam emails, leading to a more efficient and enjoyable email experience.
Challenges in managing blacklists
Despite their effectiveness, managing blacklists presents several challenges:
- False positives – legitimate emails can sometimes be mistakenly blacklisted, disrupting communication and causing inconvenience. Regular reviews and appeals processes are necessary to address these issues.
- Dynamic threat landscape – the constantly evolving nature of spam and cyber threats requires blacklists to be frequently updated. Stale or outdated blacklists may fail to protect against new threats.
- Resource allocation – maintaining and updating blacklists requires significant resources, including computing power, storage, and human oversight.
- Performance impact – implementing and managing large blacklists can impact system performance, necessitating optimization strategies to balance security and efficiency.
Techniques for effective blacklist management
To address these challenges, organizations employ several techniques for effective blacklist management:
- Regular updates – ensuring that blacklists are regularly updated with the latest data helps maintain their effectiveness against current threats. This involves automated processes for real-time updates and periodic manual reviews.
- Whitelisting – creating exceptions for trusted entities that might be wrongly blacklisted helps avoid disruption of legitimate activities. Whitelisting involves adding known safe entities to a list that bypasses the blacklist filters.
- Monitoring and feedback – continuously monitoring the performance and accuracy of blacklists helps minimize false positives and negatives. Incorporating feedback from users and other sources helps refine and improve blacklist accuracy.
- Automated tools and AI – utilizing automated tools and artificial intelligence to manage blacklists efficiently. These technologies help detect and add new threats to the blacklist, remove outdated entries, and integrate with other security systems.
- Threat intelligence sharing – collaborating with other organizations and cybersecurity communities to share information about new threats and blacklisted entities. This helps build a robust defense against emerging threats.
- Dynamic blacklisting – implementing dynamic blacklisting that can adapt to changing threat landscapes in real time. This approach allows blacklists to automatically adjust based on new threat intelligence, reducing the time lag between the emergence of a threat and its inclusion in the blacklist.
- Integration with other security measures – combining blacklists with other security frameworks, such as firewalls, intrusion detection systems, and threat intelligence platforms, to enhance overall security posture.
Future of blacklists
As cyber threats become more sophisticated, the methods for maintaining and utilizing blacklists will need to evolve. Future trends in blacklist management may include:
- AI and machine learning – utilizing advanced algorithms to better detect and respond to threats in real time. AI can analyze patterns and behaviors associated with malicious activities, enhancing the accuracy and effectiveness of blacklists.
- Collaborative blacklisting – increased collaboration between organizations to share threat data and improve the accuracy and comprehensiveness of blacklists. This approach can help identify new threats that may not be captured by a single source.
- Enhanced integration – integrating blacklists with other security measures, such as threat intelligence platforms and automated response systems, to provide a multi-layered defense against cyber threats.
- Real-time adaptation – implementing blacklists that can adapt in real-time to the changing threat landscape. Dynamic blacklists can automatically adjust based on new threat intelligence, reducing the time lag between the emergence of a threat and its inclusion in the blacklist.
Bayesian filters – using statistical methods to identify spam
Bayesian filters are a sophisticated method for identifying spam emails using statistical techniques. Named after the mathematician Thomas Bayes, this approach relies on Bayesian probability to determine the likelihood that an email is spam based on the presence of certain words and phrases.
How Bayesian Filters Work
The core principle behind Bayesian filters is to analyze the content of incoming emails and compare the frequency of specific words and phrases to a pre-compiled database of known spam and legitimate emails. Here’s a detailed look at the steps involved:
- Training the filter – initially, the filter is trained with a set of known spam and non-spam (ham) emails. Each word in the email is assigned a probability score based on its frequency in spam versus ham emails.
- Probability calculation – when a new email arrives, the filter calculates the probability of the email being spam. This is done by examining the words in the email and using the pre-determined probabilities from the training phase. The overall probability is computed using Bayes’ theorem.
- Threshold setting – the filter then compares the calculated probability to a predefined threshold. If the probability exceeds this threshold, the email is classified as spam. Otherwise, it is considered legitimate.
Advantages of Bayesian Filters
Bayesian filters offer several advantages in spam detection:
High accuracy – by learning from actual spam and ham emails, Bayesian filters can achieve high accuracy rates in identifying spam. Bayesian filters achieve high accuracy because they are trained on extensive datasets of spam and ham emails. By analyzing these datasets, the filters can assign probabilities to words and phrases, allowing them to accurately distinguish between legitimate and spam emails.
Example of Bayesian filters – consider an email filter trained on a dataset of 10,000 emails, half of which are spam and half are legitimate (ham). The filter learns that the word “lottery” appears in 80% of spam emails and only 5% of ham emails. If a new email contains the word “lottery,” the filter calculates a high probability that the email is spam. This results in a high accuracy rate because the filter relies on real data and probabilities.
Adaptive learning – Bayesian filters continuously learn and adapt to new spam tactics, making them effective against evolving spam techniques. So they try to continually update their databases with new examples, these filters stay effective even as spam techniques change.
Another example: Spammers often change their tactics to bypass filters, such as altering common phrases or using new tricks. A Bayesian filter can adapt by continuously updating its database with new spam examples. For instance, if spammers start using “free gift” instead of “lottery,” the filter will recognize this new pattern after processing several spam emails with the phrase “free gift” and adjust its probabilities accordingly.
Context awareness – context awareness allows Bayesian filters to consider the overall context of an email rather than just individual keywords. This reduces the chances of false positives, where legitimate emails are mistakenly marked as spam.
Example: A simple keyword-based filter might block any email containing the word “sale,” which could result in false positives like blocking legitimate promotional emails from trusted retailers. A Bayesian filter, however, examines the context and the probability of various words appearing together. If the word “sale” appears alongside “limited time offer” and “click here” in multiple spam emails, the filter assigns a higher spam probability. Conversely, if “sale” appears in emails from known retailers with phrases like “holiday season,” the filter is less likely to classify it as spam.
Legislative measures to reduce and penalize spam
In addition to technological solutions, legislative measures were introduced to combat spam. Notable examples include:
- CAN-SPAM Act (2003) – a U.S. law that sets rules for commercial emails, establishes requirements for commercial messages and provides penalties for violations.
- GDPR (General Data Protection Regulation) – European regulation that includes provisions on electronic communications and imposes strict requirements on consent and data protection.
CAN-SPAM Act (2003)
The CAN-SPAM Act, short for “Controlling the Assault of Non-Solicited Pornography And Marketing Act,” was enacted in the United States in 2003. This law sets the rules for commercial emails and provides recipients with the right to have emails stopped from being sent to them. The CAN-SPAM Act includes several key provisions:
- Unsubscribe mechanism – requires that all commercial emails include a clear and conspicuous way for recipients to opt out of future emails. Senders must honor opt-out requests within 10 business days.
- Content requirements – commercial emails must include a valid physical postal address of the sender. Additionally, subject lines must accurately reflect the content of the email, and emails must be clearly identified as advertisements.
- Penalties – violations of the CAN-SPAM Act can result in penalties of up to $43,792 per violation. The law also allows for criminal penalties for certain types of offenses, such as the use of fraudulent information.
GDPR (General Data Protection Regulation)
The GDPR is a comprehensive data protection regulation that came into effect in the European Union in May 2018. While it primarily focuses on data protection and privacy, it also includes provisions related to electronic communications and spam. Key aspects of GDPR relevant to spam include:
- Consent – the GDPR requires explicit consent from individuals before their data can be used for marketing purposes. This means that businesses must obtain clear and affirmative consent before sending marketing emails.
- Right to withdraw consent – individuals have the right to withdraw their consent at any time. Businesses must provide an easy way for recipients to opt out of marketing communications.
- Penalties – non-compliance with GDPR can result in significant fines. The maximum fine for serious infringements is up to 20 million euros or 4% of the company’s total global turnover, whichever is higher.
- Transparency and information – businesses must be transparent about how they collect, use, and store personal data. They must provide individuals with information about the purposes of data processing and their rights under GDPR.
Other notable legislative measures
Canada’s Anti-spam Legislation (CASL) – Enacted in 2014, CASL is one of the strictest anti-spam laws in the world. It requires businesses to obtain explicit consent before sending commercial electronic messages and includes severe penalties for non-compliance.
Australia’s Spam Act (2003) – this law regulates commercial email and SMS marketing. It requires businesses to obtain consent, provide a functional unsubscribe mechanism, and include accurate sender information.
UK’s Privacy and Electronic Communications Regulations (PECR) – PECR complements the GDPR and provides specific rules for electronic communications, including email marketing, SMS marketing, and the use of cookies.
Also about legislations covering the digital environment, see the article: Comparing Data Privacy Laws and Frameworks: An Examination of GDPR, CCPA, TCF 2.0, CPRA, VCDPA, CPA, CTDPA, and UCPA.
Advancements in spam techniques – anti-spam technologies
As anti-spam technologies improved, spammers adapted by developing more sophisticated methods to bypass filters. These techniques include:
- Obfuscation – using deliberate misspellings or non-standard characters to evade keyword-based filters.
- Image-based spam – embedding text within images to prevent text-based filters from detecting spammy content.
- Phishing – crafting emails that appear to be from legitimate sources to trick recipients into providing sensitive information.
- Spoofing – disguising the sender’s address to make the email appear to come from a trusted source.
- Snowshoe spam – distributing spam from a large number of IP addresses to avoid detection.
- Botnets – using networks of infected computers to send spam emails, making it harder to trace the origin.
- Dynamic content – changing the content of spam messages slightly for each recipient to avoid detection by filters that look for identical messages.
- PDF and attachment spam – including spam content in PDF files or other attachments to bypass text-based filters.
- Social engineering – using psychological manipulation to trick recipients into divulging confidential information.
- Malware distribution – including links or attachments that install malware on the recipient’s device.
- Backscatter spam – exploiting automated bounce-back messages from mail servers to send spam.
- Domain generation algorithms – using algorithms to create new domains rapidly, making it difficult for blacklists to keep up.
- Link hiding – using URL shorteners or other techniques to disguise the true destination of links in spam emails.
- Content injection – compromising legitimate websites to inject spammy content or links, making the source appear trustworthy.
- Time-based evasion – sending spam during off-peak hours to avoid detection by filters that are less active during these times.
- Hexadecimal encoding – encoding parts of the spam message in hexadecimal to avoid keyword detection.
- Homoglyph attacks – using characters that look similar to legitimate ones to deceive recipients (e.g., replacing ‘O’ with ‘0’).
Rise of botnets
Botnets, networks of compromised computers controlled by malicious actors, have played a significant role in the spread of spam. Botnets can send spam emails on a massive scale, making it difficult to trace the origin of the messages. They also facilitate other cybercrimes, such as distributed denial-of-service (DDoS) attacks and the spread of malware.
Impact of spam
- Economic costs – spam imposes substantial economic costs on businesses and individuals. These costs include:
- Bandwidth usage – the sheer volume of spam consumes significant bandwidth, increasing operational costs for internet service providers (ISPs) and businesses.
- Increased infrastructure costs – ISPs and businesses must invest in additional infrastructure to handle the extra load caused by spam traffic.
- Data transfer fees – companies may incur additional fees for data transfer and storage due to the high volume of spam emails.
- Productivity loss – employees waste time sifting through spam emails, reducing overall productivity.
- Time management – employees spend valuable time identifying and deleting spam, which could be used for productive work.
- Email filtering – organizations must invest in robust email filtering systems and spend time managing and maintaining these systems to keep spam at bay.
- Security risks – spam often contains malware or phishing attempts, posing security threats that can result in data breaches and financial losses.
- Malware infections – spam emails can deliver malicious software that compromises system integrity, leading to costly remediation efforts.
- Phishing attacks – spam emails designed to deceive recipients into divulging personal or financial information can lead to identity theft and financial fraud.
- Data breaches – successful phishing attacks or malware infections can result in significant data breaches, leading to regulatory fines, legal fees, and damage to an organization’s reputation.
- Operational costs – the need to continuously update and manage anti-spam technologies incurs ongoing operational expenses.
- Software and hardware investments – businesses must invest in anti-spam software, hardware, and services to protect their systems.
- Maintenance and updates – keeping anti-spam solutions effective requires regular maintenance and updates, which involves additional costs.
- User frustration – constant exposure to spam can lead to user frustration and dissatisfaction.
- Customer support burden – increased customer support requests related to spam issues can strain resources and increase costs.
- User experience degradation – a poor email experience due to excessive spam can lead to decreased user engagement and loyalty.
- Bandwidth usage – the sheer volume of spam consumes significant bandwidth, increasing operational costs for internet service providers (ISPs) and businesses.
- Environmental impact – the energy required to process and filter spam emails contributes to the carbon footprint of digital communication. Data centers and email servers consume electricity, and the additional load imposed by spam exacerbates this consumption.
- Social impact – spam can lead to decreased trust in email communication and online services.
Countermeasures against spam
Technical solutions
Various technical solutions have been developed to combat spam, including:
- Spam filters – software that uses algorithms to detect and filter out spam emails based on content analysis, sender reputation, and other criteria.
- Blacklist and whitelist – lists of known spam sources (blacklist) and trusted senders (whitelist) to help filter email traffic.
- CAPTCHAs – challenges that differentiate between human users and automated bots to prevent automated spam submissions.
- Bayesian filters – statistical methods to identify spam based on the probability of certain words and phrases appearing in spam emails.
- Content filters – analyzing the content of emails to identify and filter out spam based on keywords and patterns.
- DKIM (DomainKeys Identified Mail) – email authentication method that allows the receiver to check that an email claimed to have come from a specific domain was indeed authorized by the owner of that domain.
- SPF (Sender Policy Framework) – an email authentication method designed to detect forging sender addresses during the delivery of the email.
- DMARC (Domain-based Message Authentication, Reporting, and Conformance) – an email authentication protocol that allows the sender to indicate that their emails are protected by SPF and DKIM, and tells the receiver what to do if neither of those authentication methods passes.
- Heuristics-based filters – use a set of predefined rules to detect spam characteristics.
- Challenge-response systems – require unknown senders to verify their identity before their email is delivered.
- Greylisting – temporarily rejects email from unknown senders until they attempt to resend the message, as spammers typically do not retry sending.
User practices
Educating users about best practices can significantly reduce the impact of spam:
- Email hygiene – encouraging users to avoid posting their email addresses publicly and to use disposable email addresses for non-essential communications.
- Awareness training – teaching users how to recognize phishing attempts and spam emails.
- Reporting spam – advising users to report spam to their email provider to help improve spam detection algorithms.
Industry efforts
Industry organizations and ISPs play a critical role in combating spam. These efforts include:
- Email authentication standards – protocols such as SPF (Sender Policy Framework), DKIM (DomainKeys Identified Mail), and DMARC (Domain-based Message Authentication, Reporting, and Conformance) help verify the authenticity of email senders and prevent spoofing.
- Collaboration and information sharing – organizations collaborate to share information about emerging spam threats and develop joint strategies to combat them.
- Developing best practices – industry groups often create and promote best practices for email marketing and communication to ensure compliance with legal and ethical standards. This helps in reducing the amount of unsolicited and potentially harmful emails.
- Research and development – continuous investment in research and development (R&D) is essential for advancing anti-spam technologies and methodologies. This ongoing effort focuses on creating innovative solutions that can more effectively detect and block spam. Key areas of R&D include machine learning, artificial intelligence (AI), and other cutting-edge technologies. Here’s a deeper look into how R&D contributes to combating spam, with examples of its applications.
- Machine learning and AI – machine learning and AI play a pivotal role in the evolution of anti-spam technologies. These systems can analyze vast amounts of data to identify patterns and anomalies associated with spam. By continuously learning from new data, machine learning algorithms can adapt to new spam tactics in real time.
- For example – Google’s Gmail uses machine learning to filter out spam emails. The AI models analyze millions of emails daily, learning to distinguish between legitimate emails and spam based on various features such as email content, sender behavior, and user interactions.
- Natural language processing (NLP) – NLP is used to understand and interpret the content of emails. Advanced NLP algorithms can detect subtle differences between legitimate and spam messages by analyzing the context and structure of the text.
- For example – an anti-spam system might use NLP to analyze the language used in emails. Spam emails often use specific phrasing or keywords that legitimate emails do not. By understanding these linguistic patterns, the system can more accurately filter out spam.
- Behavioral analysis – behavioral analysis involves studying the behavior of email senders and recipients to detect anomalies that may indicate spam. This includes monitoring sending patterns, frequency, and recipient engagement.
- For example – if an email account suddenly starts sending a high volume of emails to random addresses, this could be flagged as suspicious behavior. Behavioral analysis helps to detect such anomalies and take preventive actions.
- Heuristic analysis – heuristic analysis uses a set of predefined rules to identify spam. These rules are based on known characteristics of spam emails, such as certain keywords, email formats, and header information.
- For example – a heuristic rule might flag emails containing words like “free,” “win,” or “urgent” in the subject line, as these are commonly used in spam messages.
- Advanced Encryption and Authentication – R&D in encryption and authentication technologies aims to ensure that emails are sent and received securely. Techniques like SPF, DKIM, and DMARC help verify the authenticity of email senders and prevent spoofing.
- For example – implementing DKIM ensures that an email’s content has not been altered in transit, as it is digitally signed by the sender. If the signature doesn’t match, the email can be flagged as suspicious.
- Image and attachment analysis – spammers often use images or attachments to bypass text-based filters. Advanced R&D focuses on developing technologies to scan and analyze these elements for signs of spam.
- For example – anti-spam systems might use optical character recognition (OCR) to extract text from images and check it against known spam indicators. Similarly, attachments can be scanned for malicious code or suspicious file types.
- Collaboration and information sharing – continuous R&D encourages collaboration between different organizations and industries. Sharing threat intelligence and research findings helps create a more robust defense against spam.
- For example – an email service provider might collaborate with cybersecurity firms to share data on emerging spam threats. This shared knowledge can lead to the development of more effective anti-spam technologies.
- User feedback and crowdsourcing – incorporating user feedback and crowdsourcing allows for real-time updates and improvements to anti-spam systems. Users can report spam emails, which are then analyzed to refine detection algorithms.
- For example – a user might mark an email as spam, prompting the system to analyze its content and sender information. This data helps improve the accuracy of the spam filters for future emails.
- Continuous improvement and testing – R&D is an ongoing process that involves continuous improvement and testing of anti-spam technologies. This ensures that the systems remain effective against new and evolving spam tactics.
- For example – regular updates and patches are released for anti-spam software to address vulnerabilities and improve detection capabilities. These updates are based on the latest research and threat intelligence.
- Machine learning and AI – machine learning and AI play a pivotal role in the evolution of anti-spam technologies. These systems can analyze vast amounts of data to identify patterns and anomalies associated with spam. By continuously learning from new data, machine learning algorithms can adapt to new spam tactics in real time.
- Consumer education – providing resources and educational materials to help users understand the dangers of spam and how to protect themselves. This includes guides on recognizing phishing attempts and securing personal information.
- Regulatory compliance – ensuring that industry practices comply with relevant laws and regulations, such as the CAN-SPAM Act, GDPR, and CASL. This helps in maintaining legal standards and protecting consumer rights.
E-mail authentication standards
Mail authentication standards, including SPF (Sender Policy Framework), DKIM (DomainKeys Identified Mail), and DMARC (Domain-based Message Authentication, Reporting, and Conformance), play crucial roles in verifying the authenticity of email senders and preventing email spoofing. Here’s a detailed look at how these protocols work and the techniques they use:
SPF (Sender Policy Framework)
SPF is a protocol that allows domain owners to specify which mail servers are permitted to send emails on behalf of their domain. This is done by adding a DNS (Domain Name System) record that lists the authorized IP addresses. When an email is received, the receiving mail server checks the SPF record of the sender’s domain to verify that the email comes from an authorized server.
How SPF works:
- DNS record creation – domain owners create an SPF record in their DNS settings, listing all the IP addresses or hostnames authorized to send emails.
- Email sending – when an email is sent, the sending mail server includes the domain of the sender in the email headers.
- SPF check – the receiving mail server queries the DNS for the SPF record of the sender’s domain.
- Verification – the receiving server compares the sending IP address with the list of authorized IP addresses in the SPF record.
- Action – based on the SPF check result, the receiving server decides to accept, reject, or mark the email as suspicious.
DKIM (DomainKeys Identified Mail)
DKIM is an email authentication method that allows the sender to sign their emails with a cryptographic signature. This signature is added to the email headers and is verified by the receiving server to ensure the email has not been tampered with during transit.
How DKIM works:
- Private key signing – the sender’s mail server signs the email with a private key, adding a DKIM-Signature header to the email.
- DNS Record – the sender’s domain publishes a public key in the DNS, which corresponds to the private key used to sign the emails.
- Email sending – the signed email is sent to the recipient.
- DKIM Check – The receiving mail server retrieves the public key from the sender’s DNS and uses it to verify the DKIM signature.
- Verification – If the signature is valid and matches the public key, the email is confirmed to be authentic and untampered.
DMARC (Domain-based Message Authentication, Reporting, and Conformance)
DMARC builds on SPF and DKIM by providing a way for domain owners to publish policies on how to handle emails that fail SPF or DKIM checks. It also allows domain owners to receive reports on email authentication results.
How DMARC works:
- Policy publication – domain owners publish a DMARC policy in their DNS records, specifying how to handle emails that fail SPF or DKIM checks (e.g., reject, quarantine, or allow).
- Email sending – emails are sent as usual with SPF and DKIM checks in place.
- DMARC check – the receiving mail server performs SPF and DKIM checks and then applies the DMARC policy based on the results.
- Action – the receiving server takes action based on the DMARC policy (e.g., rejecting or quarantining emails that fail checks).
- Reporting – the receiving server generates DMARC reports and sends them to the domain owner, providing insight into email authentication results and any issues detected.
Techniques used in email authentication:
- DNS records – utilized for publishing SPF, DKIM, and DMARC policies.
- Cryptographic signatures – employed in DKIM to ensure email integrity.
- Policy enforcement – DMARC policies dictate how to handle authentication failures.
- Reporting and feedback – DMARC reports provide visibility into authentication outcomes, aiding in the identification and resolution of issues.
Challenges in spam prevention
Despite significant progress, several challenges remain in the fight against spam:
- False positives – spam filters sometimes incorrectly identify legitimate emails as spam, causing important messages to be missed. Balancing the accuracy of spam detection with the risk of false positives is a continuous challenge.
- Evasion techniques – spammers constantly develop new techniques to evade detection, requiring ongoing adaptation and improvement of spam filtering technologies.
- Global maturity of spam – spam often originates from jurisdictions with lax or unenforced regulations, complicating enforcement efforts. International cooperation is essential to address this issue effectively.
- User education – educating users about the risks of spam and best practices for avoiding it is crucial. Many spam incidents result from user actions, such as clicking on suspicious links or providing personal information to unverified sources.
The future of spam
As technology evolves, so do the methods and strategies for combating spam. The future of spam prevention and detection is shaped by advancements in various fields, including artificial intelligence, blockchain technology, regulatory changes, and user-centric approaches, among many other innovative solutions. These developments aim to enhance the accuracy, efficiency, and comprehensiveness of anti-spam measures, ensuring a more secure and trustworthy online environment. By leveraging these advancements, the battle against spam can be more effectively fought, providing a safer digital experience for all users.
- AI and machine learning – advancements in artificial intelligence (AI) and machine learning (ML) hold promise for more effective spam detection and prevention. These technologies can analyze vast amounts of data to identify patterns and predict spam behavior more accurately.
- Blockchain technology – blockchain technology has the potential to enhance email security and reduce spam by providing a decentralized and tamper-proof way to verify the authenticity of email senders.
- More strict regulations – as the digital landscape evolves, governments may implement stricter regulations and penalties to deter spamming activities. This could include higher fines, increased enforcement, and more stringent data protection requirements.
- User-centric approaches – future anti-spam measures may focus more on user-centric approaches, empowering individuals with tools and knowledge to protect themselves from spam. This could involve more intuitive email clients, enhanced user education, and personalized spam filtering settings.
- Collaborative efforts – the fight against spam will likely see increased collaboration between organizations, governments, and technology providers. Sharing threat intelligence and best practices can help create a unified front against spammers, making it more challenging for them to operate.
- Advanced threat detection – with continuous investment in research and development, advanced threat detection technologies will play a crucial role in combating spam. Techniques such as behavioral analysis, real-time monitoring, and predictive analytics will become more sophisticated, enabling faster identification and response to spam threats.
- Integration with other security measures – combining spam prevention with other cybersecurity measures will provide a more comprehensive approach to protecting users. Integrating spam filters with firewalls, intrusion detection systems (IDS), and endpoint security solutions can create a multi-layered defense mechanism, enhancing overall security.
- Enhanced email protocols – future developments in email protocols may include enhanced security features designed to prevent spam. Innovations such as more robust email authentication standards, encrypted email communication, and improved sender verification processes will help create a more secure email ecosystem.
- Greater focus on mobile security – as mobile devices continue to dominate internet usage, anti-spam measures will need to adapt to the unique challenges posed by mobile platforms. Mobile-focused spam filters, secure messaging apps, and enhanced mobile email security features will be critical in protecting users from spam on their smartphones and tablets.
Was this article helpful?
Support us to keep up the good work and to provide you even better content. Your donations will be used to help students get access to quality content for free and pay our contributors’ salaries, who work hard to create this website content! Thank you for all your support!
Reaction to comment: Cancel reply