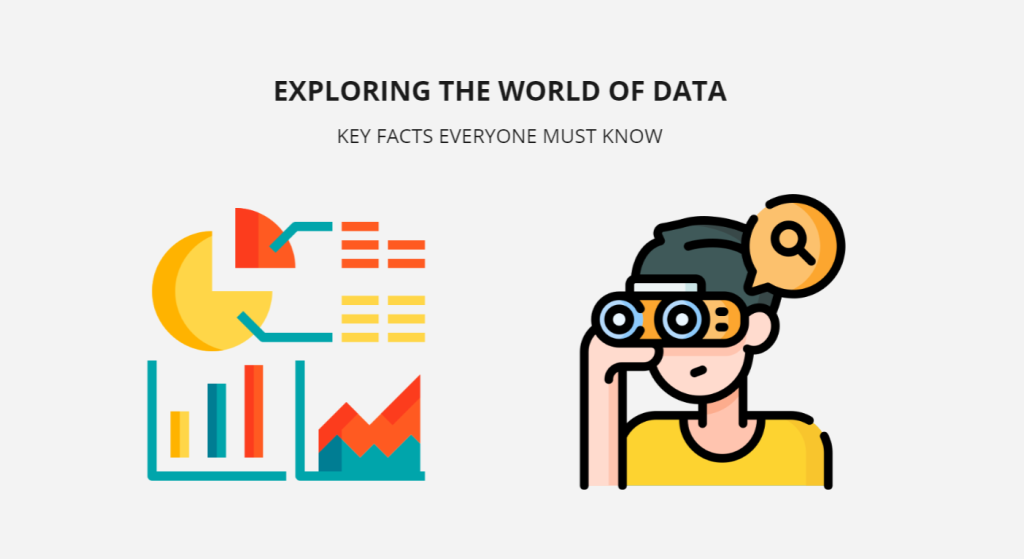
Exploring the World of Data: Key Facts Everyone Must Know
Data plays a vital role in the success of businesses that operate online, as it serves as a foundation for customer service and provides insights into customer preferences, feedback, and internal operations. Understanding the significance of data in the business world can provide a deeper understanding of how modern companies leverage it to achieve success. Therefore, this article presents 10 facts that will give you a better overview of the data.
Fact No. 1: Data is subjective
When you examine an analysis, a graph, or the rows in a table of raw data, you create your interpretation of what you see. The evidence in front of you is not based on any objective facts.
This may easily turn into an ontological debate, which is OK. The truth is that both data quality and analysis are not static.
A single set of data can switch from being worthless to being very valuable without a single piece of information changing in any way.
Fact No. 2: Data is a continuous process
Keep in mind that handling data is a process that requires more than one project. Your business has to be aware of the upstream and downstream effects of all the data wrangling going on within (and outside) its walls from a regulatory perspective.
But it goes beyond that. Every second, your business generates ludicrous volumes of data. You need a mechanism in place to effectively maintain the data pipelines inside your firm, and you need to be able to respond to variations thereof (as things are continually changing).
Fact No. 3: Data is not active
People frequently say things like “The data demonstrate that…” or “The data clearly states that…” while presenting data. Even though I understand what they are trying to say, it is still a semantic justification.
Data is not capable of performing any actions or tasks. It is a passive medium that may be exploited, wrangled, managed, sculpted, and shaped to give proof or justification for, or even a diversion from, whatever the presenter is attempting to say.
Fact No. 4: Data is limitless
The significance of this fact only grows as technology advances year after year.
You cannot possibly know all the information. It is philosophically impossible, in addition to being technically infeasible. Therefore, a boundary needs to be established. And it is crucial to comprehend the position of this line’s plot. When presenting your data set as evidence with any type of representational capabilities, you must be aware of its limitations. To maintain the results’ objectivity and reproducibility, you must be able to communicate these limits when asked to do so.
Fact No. 5: Data cannot stand silos
For some strange reason, many businesses still view data as something that can be left to a single job title (analysis, data engineer, scientist), while the rest of the organisation ignores (and neglects) the data pipeline’s broad reach.
The organisation depends on its data to function. It is indifferent to job titles. It does not care if you have a matrix organisation, flat hierarchies, or limitless PTO.
You must be aware of every area of your business where data is being gathered and processed, and you must regularly examine and audit these processes.
Fact No. 6: Tools cannot determine how your organisation operates
Many data platforms have strict guidelines. They force the organisation to adopt schemas that might not be advantageous to the company’s business cases but instead serve solely to ensure that the analytics platform predictably processes the data.
In general, monolithic, generalised schemas are disadvantageous. The company is compelled to adapt to the analytics platform instead of the platform being tailored to the company’s needs.
I can still recall thinking for many hours about how I might “trick” Google Analytics into analysing an Add To Cart event on a website without a shopping cart so that I could use the e-commerce report suite. No one should be forced to complete this task.
Fact No. 7: Data can be overlooked or neglected
It is a mistake to say you are “data-driven.” Avoid falling for it! Based on my many years of experience, the vast majority of businesses operate with data that is entirely misinterpreted and whose baseline quality is just ridiculously low (but keep in mind Fact No. 1).
If the analysis suggests A, and this is supported by experimentation, thorough testing, and the most reliable data set you will ever see, but your intuition suggests B, feel free to follow it. You can ignore the data.
Although Fact No. 3 should be kept in mind, there is no categorical imperative for you to act under the data.
However, for the business case to make sense, you must be able to support your decision with evidence that is at least as effective as following the advice provided by the data analysis.
You cannot just throw a tantrum and ignore the facts because you believe it is your divine right to jump over the planet’s edge to make a pointless argument. You have to be able to create a business case for your decision and persuade your colleagues that the risk is worthwhile.
Fact No. 8: Data is a secondary result of other processes
Okay, so this is not always the case (surprise!), but it is still important in the context of analytics and digital marketing.
Applications, websites, and services with a primary focus on data generation have an extremely limited number of actual features.
Instead, most of the time as analysts, we make use of already-existing features and, as a secondary outcome, add data collection.
A checkout form’s central objective is not to increase conversions. No, the primary focus of it is to encourage sales. The conversion ping is only a secondary result of this process.
As analysts, we frequently forget that most of the time, our businesses, clients, developers, or even marketers do not care that much about data collection since we are too focused on the significance of our job. They only desire that the feature fulfil its intended function.
Data engineering jobs are frequently given lower priority as a result. It is unfortunate, but it is also true.
Clarifying the significance of these side effects is also something that the data scientist must do. The data engineer’s (or analyst’s) position frequently involves consulting since they have to show others how these side effects might truly be worth the time and resource commitment rather than just being development overhead.
Fact No. 9: Data can be complex and hard to manage
All of my presentations for years and years concluded with a slide that read:
Data is challenging. Data quality is not purchased; it is earned.
This, in my opinion, is still vital. Particularly during the COVID-19 epidemic, more and more people were exposed to charts, analyses, and data interpretations that were incorrect.
I hope people realise how challenging it is to not only gather data but to comprehend how that data will be processed, how that data will affect downstream processes, how that data will be regulated, and how to display that data in a meaningful way.
I want people to realise that “ML” and “AI” are more than simply fancy abbreviations. Machine learning and artificial intelligence algorithms require fine-tuning and a human component with the knowledge (and guts) to initiate the processes.
Working with data is more challenging than ever. There are still no fast cuts; data quality must be achieved via effort, passion, and a strong character.
Fact No. 10: Valuable insights are not always easy to obtain, and it’s alright
I believe that many analysts think and act like John Nash in A Beautiful Mind, looking at a data set with the expectation that patterns would suddenly emerge and spark a brilliant discovery that will radically transform their business.
Well, either you will have to wait a while, or you are not doing your job properly.
In evolutionary biology, there is a wonderful concept known as punctuated equilibrium. Most of the evolution, according to this, actually occurs at a slow, constant pace. But once in a while, significant changes bring about a chaotic, quick transformation.
Many analysts, in my opinion, fail to see this and instead try to bring these changes using new tools, collection methods, and schemas to “get results.”
However, a lot of what we do in analytics is focused on careful observation and delivering consistent data for other processes to use.
Was this article helpful?
Support us to keep up the good work and to provide you even better content. Your donations will be used to help students get access to quality content for free and pay our contributors’ salaries, who work hard to create this website content! Thank you for all your support!
Reaction to comment: Cancel reply