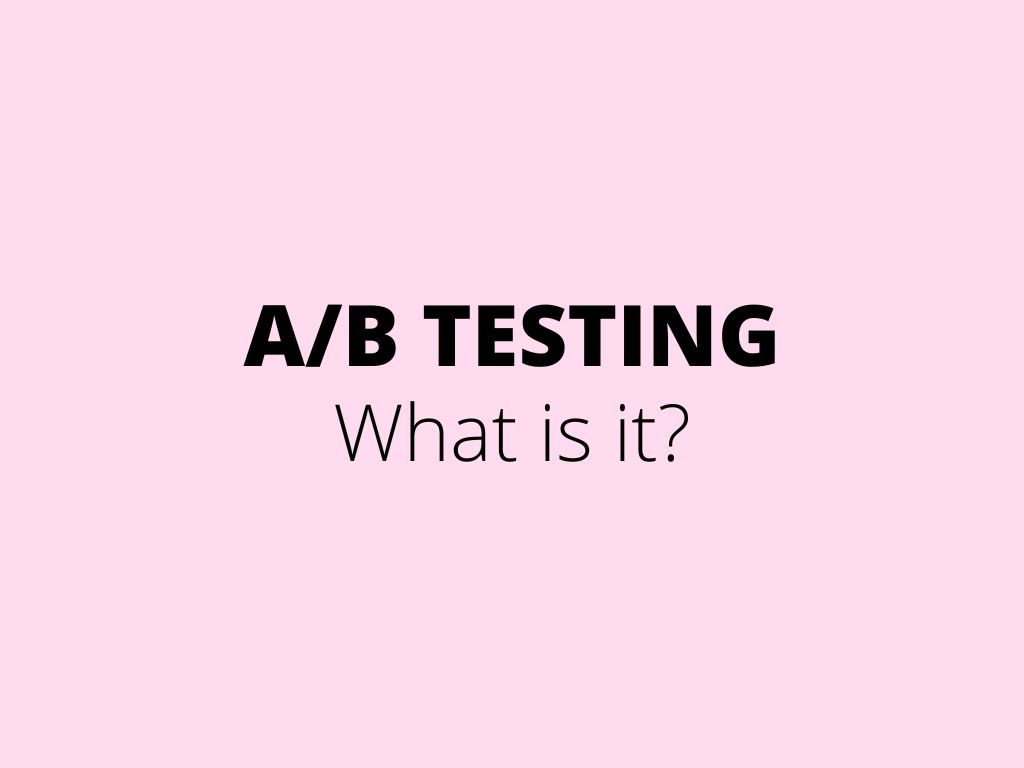
A/B testing – what is it?
Let’s start with the general A/B testing definition.
A/B testing, also known as split testing or bucket testing, is a method of comparing two versions of content to determine which one performs better. By testing a control version (A) against a variant (B), measures success based on key metrics such as conversion rates or user engagement. This technique is widely used in both B2B and B2C marketing.
Common applications include:
- Website A/B testing – splits traffic between two versions of a webpage (e.g., copy, images, design, calls to action) to see which one results in higher conversions or desired actions.
- Email marketing A/B testing – divides email recipients into two segments to test different subject lines, images, or calls to action, aiming to identify which version achieves a higher open rate.
- Content selection – compares editor-selected content with algorithmically selected content based on user behavior to see which generates more engagement.
A/B testing is crucial for optimizing customer experience (CX) and can be extended to A/B/N testing, where “N” represents multiple variations beyond just two.
When and why to use A/B testing
A/B testing is most beneficial when implemented continuously, providing ongoing recommendations for improving performance. The nearly unlimited options for testing include:
- Emails
- Newsletters
- Advertisements
- Text messages
- Website pages
- Components on web pages
- Mobile apps
A/B testing is vital for campaign management, revealing what resonates with your audience and what doesn’t. It helps identify which elements of your marketing strategy have the most significant impact, need improvement, or should be discarded.
When to A/B test:
- Underperforming campaigns – if a digital marketing campaign or element isn’t meeting expectations, A/B testing can help isolate and address performance issues.
- Launching new elements – before launching new web pages, email campaigns, or other digital assets, proactive A/B testing can determine the most effective approach by comparing different strategies.
By consistently applying A/B testing, marketers can optimize their strategies, enhance user experiences, and achieve better results across various digital channels.
Benefits of running A/B tests on your website
Website A/B testing is an effective method to determine which tactics resonate best with your visitors. It allows you to validate your hypotheses with data, ensuring you continue using only the most effective strategies. Even if your hunch is proven wrong, it’s a positive outcome since you avoid sticking with ineffective tactics. Successful A/B tests lead to increased visitor engagement, longer site visits, and more link clicks.
Testing commonly used website components and sections help you make informed decisions that can improve not just the test page but other similar pages across your site. This process ensures consistent enhancements in user experience and site performance.
How to perform an A/B test
A/B testing involves a systematic approach to ensure reliable and actionable results. Here are the nine fundamental steps to plan and execute an A/B test:
- Measure and review the performance baseline – understand the current performance metrics of your website to establish a baseline.
- Determine the testing goal – define what you aim to achieve with the test, based on your performance baseline.
- Develop a hypothesis – formulate a hypothesis on how the changes you’re testing will improve performance.
- Identify test targets or locations – choose specific elements or pages on your website to test.
- Create the A and B versions – develop the control (A) version and the variant (B) version for comparison.
- Utilize a QA tool – use a quality assurance tool to validate that your test setup is correct.
- Execute the test – run the A/B test, ensuring both versions are equally represented to your audience.
- Track and evaluate results – use web and testing analytics to monitor performance and gather data.
- Apply learnings – analyze the results and apply the insights to improve the customer experience.
Following these steps with clear goals and a solid hypothesis helps avoid common A/B testing mistakes. The data and empirical evidence from these tests guide you in refining and enhancing your website’s performance. Continuous optimization through A/B testing leads to more engaging customer experiences, compelling content, captivating visuals, and ultimately, more effective marketing strategies. This, in turn, increases ROI and drives higher revenue.
By integrating these practices into your digital marketing efforts, you can ensure that your website consistently evolves to meet the needs and preferences of your audience, leading to sustained growth and success.
A/B testing examples
A/B testing can be applied to various digital marketing elements to optimize performance. Here are some examples of what can be tested:
- Navigation links – experiment with different placements and labeling of navigation links to see which version enhances user navigation and engagement.
- Calls-to-actions elements (CTAs) – test different cta text, colors, and placements to determine which combinations drive more conversions.
- Design/layout – compare different page layouts and design elements to see which arrangement improves user experience and interaction.
- Copy – test variations in the website copy, including length, tone, and style, to identify what resonates best with your audience.
- Content offers – evaluate different content offers, such as eBooks, whitepapers, or webinars, to see which one attracts more interest.
- Headlines – experiment with different headlines to determine which one grabs attention and encourages further reading.
- Email subject lines – test various subject lines to find which ones lead to higher open rates.
- Friendly email “from” address – see if changing the sender’s name impacts open rates and engagement.
- Images – compare different images to see which ones drive more engagement and conversions.
- Social media buttons – test the presence, placement, and design of social media buttons to see their effect on social sharing.
- Logos and taglines/slogans – experiment with different logo designs and taglines to find which combinations strengthen brand perception and recognition.
Your business goals, performance objectives, baseline data, and current marketing campaign mix will help you determine the best candidates for A/B testing.
The role of analytics in website A/B testing
Analytics is essential throughout the entire lifecycle of an A/B test, from planning to execution and performance evaluation.
Planning stage
The development of a test hypothesis requires a strong foundation in analytics. Understanding current performance and traffic levels is crucial. Key data points provided by your analytics system during the planning process include:
- Traffic – page views and unique visitors to the page or component being tested
- Engagement – metrics such as time spent on the page, pages per visit, and bounce rate
- Conversions – data on clicks, registrations, and fallout rates
- Performance trends – trends over time to provide context for current performance level
Without a solid grounding in analytics, test scenarios may be based on assumptions or personal preferences, which are often proven incorrect by testing.
Execution stage in A/B testing
Once an A/B test launches, analytics plays a central role in monitoring and validating performance metrics in real-time. A performance dashboard helps track these metrics, ensuring the test operates as expected and allows for quick responses to any anomalies or unexpected results. Adjustments can be made, and the test can be restarted if necessary, ensuring that performance data accurately reflects any changes and their timing.
The performance dashboard also helps determine the duration of the test and ensures statistical significance is achieved before making any conclusions.
Post-test analysis in A/B testing
After the test concludes, analytics are used to determine the next steps. This may involve adopting the winning version as the new standard presentation on the tested page and potentially rolling it out to similar pages. Developing a reusable analytics template to convey test results and adapting it for specific test elements can streamline the process and enhance future tests.
By leveraging A/B testing and comprehensive analytics, marketers can continuously refine their strategies, enhance user experiences, and achieve better performance across digital channels.
How to interpret A/B test results
To effectively interpret A/B test results, it’s crucial to establish clear goals during the planning phase. This helps you evaluate the outcomes, determine a winner, and update your marketing campaign or website to reflect the successful version. Often, an audience is pre-segmented with a holdout group that receives the winning version of a message.
The test results will indicate the success of one element over another based on the metrics you’ve decided to measure, such as:
- Number of visitors
- Open rates
- CTR/Click-through rates
- Signups (for newsletters, etc.)
- Subscriptions
During the test, the two elements are monitored until a statistically significant measurement is achieved.
Conversion rates can also be measured in terms of revenue. Consider sales numbers and the impact of a change on actual sales revenue. Remember, conversion rates can be captured for any measurable action, not just e-commerce sites and sales. These actions can include:
- Sales
- Leads generated/registrations submitted
- Newsletter signups
- Clicks on banner ads
- Time spent on the site
What metrics should you pay attention to when it comes to A/B testing?
The metrics to focus on depend on your hypothesis and goals. However, you should prioritize metrics that indicate audience engagement with your marketing content.
- For web pages – look at the number of unique visitors, return visitors, time spent on the page, bounce rates, and exit rates.
- For email marketing – monitor who opens the email and clicks through to your CTAs.
By focusing on these engagement metrics, you can make data-driven decisions to enhance your marketing strategies and improve overall performance.
What is multivariate testing? How is it different from A/B testing?
Multivariate testing is often discussed alongside A/B testing, so it’s important to understand its distinct characteristics and how it differs from A/B testing. Although they are related, there are significant differences between the two.
- Multivariate testing involves testing multiple elements simultaneously across one or more website pages or email campaigns to identify the combination that yields the highest conversion rate. It applies a statistical model to test various combinations of changes, leading to an overall optimized user experience and website performance.
- A/B testing, on the other hand, compares two versions of a single element to see which one performs better.
Here are some key traits of multivariate testing:
- Wide range of elements – multivariate tests involve a broad array of changes, including images, text, color, fonts, links, CTA buttons, and layout for landing pages or checkout processes. It is common for a multivariate test to exceed 50 or more combinations.
- From hypothesis to results – multivariate testing begins with a hypothesis about content changes that could improve conversion rates. These changes are broken down into individual elements to determine the most effective combinations. Whether the changes are slight or significant, they can impact the overall results.
- Conversion rates – the primary metric in multivariate testing is the conversion rate, which measures the rate at which visitors perform a desired action, such as clicking an offer or adding products to their cart. Additional metrics like revenue per order or click-through rate are also evaluated. Analytics help identify which combination of changes yields the best results based on the defined metrics.
- Continuous optimization – multivariate testing allows for continuous optimization by defining a business goal and letting the software automatically optimize the visitor experience to achieve that goal. This process ensures that the most effective combinations are used to enhance performance consistently.
In summary, while A/B testing compares two versions of a single element, multivariate testing evaluates multiple elements and their combinations simultaneously. This comprehensive approach provides deeper insights into which combinations of changes work best together to achieve the highest conversion rates and overall website optimization.
Can you run A/B and multivariate tests on iOS and Android Apps?
Yes, you can run A/B and multivariate tests on iOS and Android apps. In 2020, mobile apps accounted for $2.9 trillion in e-commerce spending, a figure expected to rise by an additional trillion by the end of 2021. This growth isn’t limited to retail and e-commerce; mobile traffic’s share of total online traffic continues to increase rapidly. In many regions, mobile phones are more accessible than laptops, making them a critical part of the customer’s buying journey. However, due to smaller screens, the cart abandonment rate is higher on mobile (87%) compared to desktops/laptops (73%).
Optimizing your mobile app experience is crucial, but it requires the right tools to account for the limitations of iOS and Android platforms. Effective A/B and multivariate testing can significantly enhance user experience and reduce cart abandonment rates.
Visitor segmentation and segment clustering in multivariate testing
Not all visitors or recipients will respond the same way to a particular experience. A key advantage of multivariate testing is the ability to identify and target different visitor segments based on how they interact with various experiences. For example, new visitors might prefer a different experience than repeat visitors, leading to better overall results. Advanced systems can automatically suggest visitor segmentation, saving time by analyzing test results against numerous visitor attributes.
Targeting different experiences for different visitor segments can substantially increase conversion rates. Segmentation can be based on a wide range of visitor attributes, from environmental factors to behaviors, and can include customer data from systems like your CRM.
When to use A/B testing vs. multivariate testing
Deciding whether to use A/B testing or multivariate testing depends on the number of elements you need to test and the complexity of their interactions.
A/B testing is ideal when you have two versions of an element to compare. It’s straightforward and easy to understand, making it a good introduction to website and campaign optimization. It helps demonstrate the measurable impact of a design change or tweak.
Multivariate testing is better suited for testing multiple elements and their combinations. It’s useful when you want to compare various combinations of images, titles, or other elements on a webpage or email. However, more options require higher traffic to achieve statistically significant results. Testing too many elements simultaneously can lead to an overwhelming number of permutations, which most websites and email campaigns cannot support due to insufficient traffic.
Was this article helpful?
Support us to keep up the good work and to provide you even better content. Your donations will be used to help students get access to quality content for free and pay our contributors’ salaries, who work hard to create this website content! Thank you for all your support!
Reaction to comment: Cancel reply